Understanding Machine Learning in personalized Marketing campaigns
- Harshal Karandikar
- Aug 21, 2024
- 4 min read
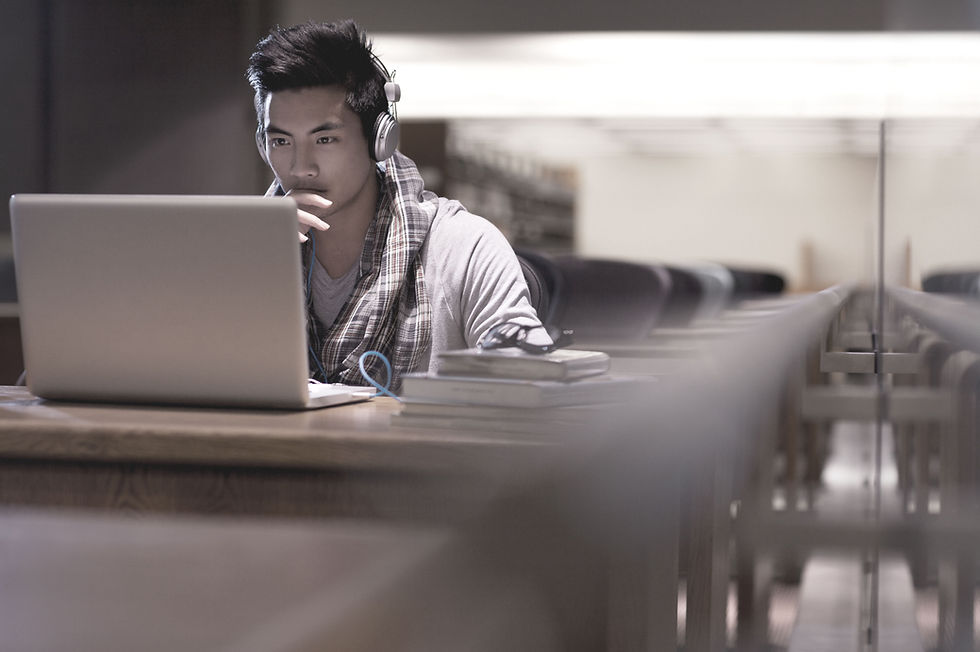
In today’s digital era, personalization has become key for successful marketing strategies. Before understanding the role of machine learning in marketing campaigns, let us have a fair understanding on Machine Learning in Marketing.
Machine Learning is arm of AI that focuses on the using data and algorithms to enable AI to replicate how human learns and thinks. In the context of marketing, ML algorithms analyze vast amounts of data to identify patterns, predict outcomes, and make decisions to boost effectiveness of marketing campaigns.
The rise of big data has given unprecedented access to marketers about consumer behavior, preferences, choices, buying patterns and interactions. And to understand this big data manually in nearly impractical, and that is where ML comes into play. By leveraging ML, marketers can process and analyze data at scale, uncovering insights that drive more targeted and personalized marketing efforts.
In today’s dynamic marketing world consumers crave for the experiences that resonates with them on personal level and this is where ML comes into play. With the help of ML marketers can deliver highly personalized marketing campaigns that cater to individuals’ preferences and behaviors.
1. Customer Segmentation: Beyond Demographics :
One of the primary applications of machine learning in personalized marketing is customer segmentation. Traditional segmentation methods often rely on broad demographics like age, gender, or location. As these factors are important but they don’t capture minute details of the modern customers. Machine learning allows free hand to marketers to move beyond these basic demographics and segment customers based on a wide range of variables, including online behavior, purchase history, social media activity, and even psychographic factors like interests and values.
For example, many customers browse popular E-commerce site for purchases and products to their cart. They wait for Big Billion Sale or other offers to make a purchase. For these kind of customers Marketer can use machine learning to identify a segment of customers to create targeted campaigns offering exclusive discounts to this segment, increasing the likelihood of conversion.
ML-driven segmentation also enables dynamic segmentation, where customer groups are continuously updated as new data is collected. This ensures that marketing efforts remain relevant and aligned with evolving consumer behaviors and preferences.
2. Predictive Analytics: Anticipating Customer Needs :
Predictive analytics is another critical component of personalized marketing, powered by ML. By analyzing historical data, ML algorithms empowers and allows marketers to predict future consumer behavior and preferences even before they arise.
For instance, an e-commerce platform might use predictive analytics to identify customers who are likely to make a repeat purchase within a specific timeframe. The platform can then send personalized recommendations or reminders to these customers, encouraging them to return and complete a purchase. This proactive approach not only boosts sales but also enhances the customer experience by providing timely and relevant content.
Predictive analytics can also be used to identify customers who may be on at-risk or on the verge of churning. By recognizing early warning signs such as, reduced engagement, very less website sessions, longer purchase intervals, less activities in adding cart, marketers can deploy targeted retention campaigns to re-engage these customers, offering incentives or addressing potential issues before they lead to churn.
3. Personalized Content and Recommendations :
Personalized content is at the heart of successful marketing campaigns, and machine learning plays a pivotal role in delivering it. ML algorithms can analyze a user’s behavior, preferences, and past interactions to generate personalized content recommendations, whether it’s products, articles, videos, or advertisements.
Consider how streaming services like Netflix or music platforms like Spotify use machine learning to create personalized recommendations for their users. By analyzing what a user has previously watched or listened to, these platforms can suggest new content that aligns with the user’s tastes, keeping them engaged and satisfied.
This same principle applies to e-commerce, where personalized product recommendations can significantly enhance the shopping experience. For example, Amazon’s recommendation engine uses machine learning to suggest products based on a user’s browsing history, previous purchases, and items that other customers with similar interests have bought. These recommendations are often displayed prominently on the homepage or during the checkout process, increasing the chances of additional purchases.
In the realm of content marketing, machine learning can help personalize email campaigns by determining the most relevant content to send to each subscriber. Instead of sending the same generic email to everyone, marketers can use ML to tailor emails to individual interests, resulting in higher open rates, click-through rates, and conversions.
4. Optimizing Ad Targeting and Spend :
Machine learning is also revolutionizing how businesses approach ad targeting and spends. Traditional ad campaigns involve a significant amount of guesswork on deciding target audiences, media and platform to use and how much to spend. ML eliminates all the above by analyzing data to determine the most effective strategies.
ML algorithms can process data from various sources, such as browsing history, social media interactions, and past purchase behavior, to identify the audiences most likely to respond positively to a specific ad. This enables marketers to deliver ads that are not only relevant but also more likely to lead to conversions.
Moreover, machine learning can optimize ad spend by dynamically adjusting bids in real-time based on performance data. If an ad is performing well, the algorithm can increase the bid to maximize exposure. Conversely, if an ad isn’t performing as expected, the algorithm can reduce the bid or shift the budget to more effective ads. This level of automation ensures that marketing budgets are used efficiently, leading to better ROI.
5. Enhancing the Customer Journey :
Machine learning isn’t just about improving individual aspects of a marketing campaign; it’s about enhancing the entire customer journey. By understanding customer behavior at every touchpoint—from initial awareness to post-purchase engagement—ML algorithms can help marketers create a seamless and personalized experience.
For example, machine learning can analyze how customers navigate a website, identifying patterns in how they move from one page to another. This data can be used to optimize the site’s layout, making it easier for users to find what they’re looking for and increasing the likelihood of a purchase.
Machine learning can also personalize the customer journey by tailoring interactions based on where the customer is in the buying process. A first-time visitor might see educational content designed to build trust, while a returning customer might be shown personalized product recommendations or loyalty offers.
コメント